About
I am a Flatiron Research Fellow, at Flatiron Institute, Center for Computational Mathematics. My general research interest lies in Bayesian computation, Bayesian modeling, machine learning, and causal inference.
Before Flatiron, I earned my Ph.D. in Statistics from Columbia University in 2021 under the supervision of Andrew Gelman. Before that, I obtained my undergraduate education from Tsinghua University in Mathematics and in Economics.
About my research
• I build scalable Bayesian for applied data problems, with a focus on probabilistic modeling and uncertainty quantification. My recent applications included lead fallout in Paris, arsenic diffusion in South Asia, Covid-19 mortality in Bangladesh, and galaxy clustering in the Universe.
• But better applied statistics needs better methodology. To that end, I design statistical and machine learning methods, with a focus on model evaluation, aggregation, causal inference and prediction under misspecification. Some ongoing progresses are on cross-validation, stacking and hierarchical stacking, and covariate imbalance.
• But complex methods further need scalable and diagnosable computing. Hence, I develop algorithms and theories for fully Bayesian and approximate computations, including importance sampling, simulated tempering and annealing, and multimodal MCMC sampling. My recent interest is on simulation-based and score-based methods for scientific computing, and the general framework of distribution aggregation flow.
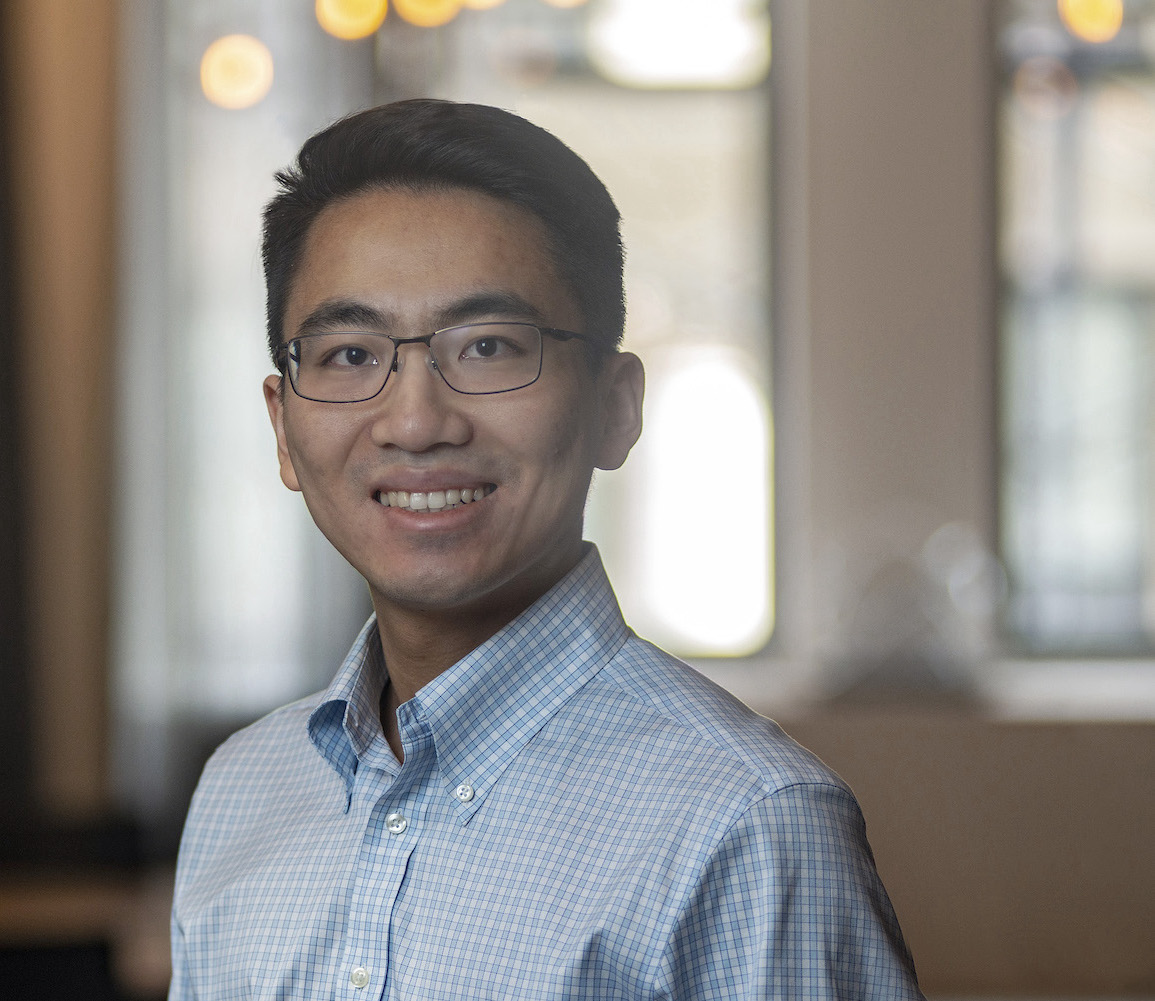
yyao@yyao.dev
646-908-6510
Twitter
Blog
Blog 2
Résumé
Google scholar
Github
Flatiron Institute, CCM
162 5th Ave
New York, NY 10010
Publications
Bayesian methodology (stacking, cross-validation, prediction, and causal)
Simulation based stacking. AISTATS.
Yuling Yao, Bruno Régaldo-Saint Blancard, Justin Domke (2024).
A cheat sheet for Bayesian prediction. arxiv.
Bertrand Clarke, Yuling Yao. (2023).
Locking and Quacking: Stacking Bayesian models predictions by log-pooling and superposition. NeurIPS ( Workshop on Score-Based Methods).
Yuling Yao, Luiz Max Carvalho, Diego Mesquita. (2022).
Make cross-validation Bayes again. NeurIPS workshop.
Yuling Yao, Aki Vehtari. (2021).
[Poster]
Toward a scalable Bayesian workflow. PhD Thesis.
Yuling Yao. (2021).
Bayesian hierarchical stacking: Some models are (somewhere) useful. Bayesian Analysis.
Yuling Yao, Gregor Pirš, Aki Vehtari, Andrew Gelman. (2021).
[Code] [Blog]
[Talk]
Holes in Bayesian statistics. Journal of Physics G.
Andrew Gelman, Yuling Yao. (2020).
Bayesian aggregation. Wiley StatsRef: Statistics Reference Online.
Yuling Yao. (2020).
Limitations of "Limitations of Bayesian leave-one-out cross-validation for model selection". Computational Brain & Behavior.
Aki Vehtari, Daniel Simpson, Yuling Yao, Andrew Gelman. (2018).
Using stacking to average Bayesian predictive distributions (with discussions and rejoinder). Bayesian Analysis.
Yuling Yao, Aki Vehtari, Daniel Simpson, Andrew Gelman. (2018).
[Code] [R package ]
Computing (MCMC, variational, importance sampling, and likelihood-free)
Discriminative calibration. Neurips.
Yuling Yao, Justin Domke. (2023).
Stacking for non-mixing Bayesian computations: The curse and blessing of
multimodal posteriors. Journal of Machine Learning Research.
Yuling Yao, Aki Vehtari, Andrew Gelman. (2022). [Code] [Blog]
[Talk]
Pareto smoothed importance sampling. Journal of Machine Learning Research
Aki Vehtari, Daniel Simpson, Andrew Gelman, Yuling Yao, Jonah Gabry. (2022+).
Adaptive path sampling in metastable posterior distributions. under review.
Yuling Yao, Collin Cademartori, Aki Vehtari, Andrew Gelman. (2020). [Package] [Blog]
Yes, but did it work?: Evaluating variational inference. International Conference on Machine Learning.
Yuling Yao, Aki Vehtari, Daniel Simpson, Andrew Gelman. (2018). [Blog]
[Code]
[Talk]
Ensemble model patching: A parameter-efficient variational Bayesian neural network.
Oscar Chang, Yuling Yao, David Williams-King, Hod Lipson. (2019).
Applied statistics (ML-for-Science, public health and survey)
SimBIG: Galaxy clustering analysis with the wavelet scattering transform. arxiv.
Bruno Régaldo-Saint Blancard, ChangHoon Hahn, Shirley Ho, Jiamin Hou, Pablo Lemos, Elena Massara, Chirag Modi, Azadeh Moradinezhad Dizgah, Liam Parker, Yuling Yao, Michael Eickenberg. (2023).
Assessment of excess mortality and household income in rural Bangladesh during the Covid-19 pandemic in 2020. JAMA Network Open.
Prabhat Barnwal*, Yuling Yao* (equal contribution), Yiqian Wang, Nishat Akter Juy, Shabib Raihan, Mohammad Ashraful Haque, Alexander van Geen. (2021).
[Media coverage]
Economic growth and happiness in China: A Bayesian multilevel age-period-cohort analysis based on the CGSS data 2005–2015. International Review of Economics and Finance.
Yu-Sung Su, Donald Lien, Yuling Yao. (2021).
Making the most of imprecise measurements: Changing patterns of arsenic concentrations in shallow wells of Bangladesh from laboratory and field data. preprint.
Yuling Yao, Rajib Mozumder, Benjamin Bostick, Brian Mailloux, Charles Harvey, Andrew Gelman, Alexander van Geen. (2021).
Bayesian workflow. preprint.
Andrew Gelman, Aki Vehtari, Daniel Simpson, Charles Margossian, Bob Carpenter, Yuling Yao, Paul-Christian Bürkner, Lauren Kennedy, Jonah Gabry, Martin Modrák. (2020).
Fallout of lead over Paris from the 2019 Notre-Dame Cathedral fire. Geohealth.
Alexander van Geen, Yuling Yao, Tyler Ellis, Andrew Gelman. (2020).
[Code]
[Media coverage (Le Monde)]
[Media coverage 2]
A Bayesian bird's eye view of ‘Replications of important results in social psychology’. Royal Society Open Science.
Maarten Marsman, Felix D Schönbrodt, Richard D Morey, Yuling Yao, Andrew Gelman, Eric-Jan Wagenmakers. (2016).
Software
I am among the developer teams of the following softwares:
LOO
An R package for efficient approximate leave-one-out cross-validation (LOO) using Pareto smoothed importance sampling (PSIS), a new procedure for regularizing importance weights.
Stan

Stan is a state-of-the-art platform for statistical modeling and high-performance statistical computation. Thousands of users rely on Stan for statistical modeling, data analysis, and prediction in the social, biological, and physical sciences, engineering, and business.
Media appearance
-
“Lead fallout from Norte Dame fire was likely overlooked,” Interviewed by State of the Planet, Columbia University news site, 9 July 2020.
Yao said, “Of course, we are measuring slightly different things, but ultimately all disagreement in scientific findings shall be validated by more data, especially when they have profound policy and public health consequences. I hope our work sheds some light in that direction.”
-
“Incendie de Notre-Dame: une nouvelle étude relance la question de l’exposition au plomb,
”
Le Monde, 11 July 2020.
Des chercheurs de l’université Columbia suggèrent que les Parisiens résidant à moins d’un kilomètre de la cathédrale auraient été exposés à des niveaux très supérieurs aux données officielles.
-
“Paris Beehives Trace Notre-Dame’s Toxic Fallout,
”
New York Times, 29 July 2020.
Scientists at Columbia University found that people living within 1,100 yards and downwind of the fire were likely to have been exposed to more lead fallout than previously announced.
- “Where COVID-19's Death Grip Slipped (Briefly),” State of the Planet, Columbia University news site, 15 November 2021.
© Yuling Yao